Top 10 Machine Learning Applications Transforming Industries
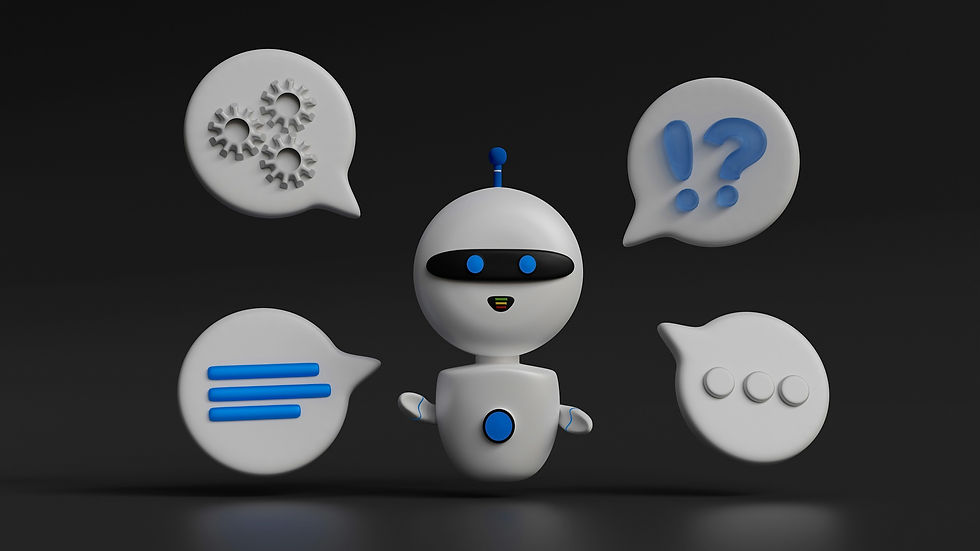
Machine learning continues to be one of the most transformative technologies across various industries, and its applications are expanding even further. Whether you're browsing your favorite streaming platform, getting personalized recommendations, or experiencing advanced healthcare diagnostics, machine learning (ML) is likely driving those experiences.
In this article, we’ll explore the top 10 machine learning applications that are shaping industries and daily life.
1. Generative AI for Content Creation
Generative AI is making waves in content creation, offering unparalleled capabilities for generating text, images, music, and video. Tools such as GPT-4, DALL·E, and similar generative models use deep learning to mimic human creativity. These models generate high-quality and innovative content with minimal human input, helping artists, marketers, and content creators produce engaging material quickly and effectively.
Applications:
Automated Writing: Generative AI can produce articles, social media posts, and reports, reducing manual effort while maintaining a human-like tone.
Image and Video Generation: From creating unique artworks to producing video content based on textual input, generative models assist creative professionals.
Music Composition: AI tools can compose music tailored to specific moods, helping artists and media professionals produce original soundtracks.
Generative AI is revolutionizing sectors like advertising, publishing, and entertainment, making creative processes faster and more efficient. With these tools, artists and content creators can focus on the quality and originality of ideas while delegating repetitive tasks to AI systems. This transformation is reshaping industries, allowing creative teams to do more with fewer resources and in less time.
2. Healthcare Diagnostics and Personalized Medicine
Machine learning is revolutionizing healthcare by providing tools that assist in medical diagnosis and personalized treatment plans. AI-powered diagnostic tools now analyze medical data such as X-rays, MRIs, and genetic data to detect diseases earlier and more accurately than ever before.
Applications:
Early Disease Detection: AI models detect conditions such as cancer, heart disease, and neurological disorders from medical images and lab results.
Predictive Analytics: Machine learning models predict patient outcomes, allowing for proactive treatment approaches.
Genomic Analysis: By analyzing patients' genetic data, AI helps in crafting personalized treatment plans, improving the effectiveness of therapies.
These advancements lead to faster diagnosis, better patient outcomes, and a shift toward preventive healthcare. For example, AI models have been able to spot abnormalities that even experienced radiologists may overlook, significantly improving early intervention measures. Personalized treatment, supported by AI, ensures that patients receive therapies specifically tailored to their health profile, making treatments more effective and minimizing adverse reactions. As AI continues to evolve in healthcare, it holds promise for reducing human error and addressing inefficiencies in the medical field.
3. Autonomous Vehicles and Smart Transportation
Autonomous vehicles are no longer a future concept, with machine learning at the heart of smart transportation systems. Self-driving cars rely heavily on machine learning algorithms to navigate safely, detect objects, and make decisions on the go.
Applications:
Autonomous Cars: Machine learning enables cars to understand and adapt to their surroundings, improving their ability to drive safely.
Smart Traffic Systems: Cities use ML to monitor traffic conditions and adjust traffic signals in real-time to reduce congestion.
Predictive Maintenance: Fleet management systems use ML to predict when vehicles will require maintenance, reducing downtime and repair costs.
This technology is transforming transportation into a safer, more efficient, and environmentally friendly system. Machine learning in smart transportation not only enhances safety but also contributes to energy efficiency by optimizing routes and traffic management. As ML-driven autonomous vehicles become more widespread, the shift could dramatically reduce accidents caused by human error, providing significant societal benefits in terms of public safety and cost savings.
4. Natural Language Processing (NLP) for Virtual Assistants
Natural Language Processing (NLP) is advancing rapidly, making virtual assistants like Siri, Alexa, and Google Assistant smarter and more responsive. NLP allows these assistants to understand not just the words you speak but the intent and context behind them, leading to more natural conversations and improved task execution.
Applications:
Personalized Assistance: Virtual assistants manage tasks such as scheduling, shopping, and answering complex queries with better understanding and efficiency.
Customer Support: NLP-powered chatbots handle customer inquiries with contextual understanding, providing solutions faster and with fewer errors.
Language Translation: Real-time translation tools use NLP to provide accurate translations during conversations, breaking down language barriers.
These tools are becoming indispensable in homes and businesses alike, simplifying everyday tasks and enhancing customer experiences. NLP advancements mean that virtual assistants are moving beyond simple commands and are now engaging in meaningful, context-rich dialogues. This progress makes interactions feel more natural and trustworthy, which is crucial as more users integrate these systems into their daily routines.
5. Fraud Detection and Cybersecurity
Cyber threats are evolving, and machine learning is essential in staying ahead of malicious activities. Fraud detection and cybersecurity systems use advanced ML models to monitor transactions, detect anomalies, and predict potential threats before they escalate into breaches.
Applications:
Real-Time Fraud Detection: Financial institutions use ML to detect fraudulent transactions by analyzing patterns and behaviors in real-time.
Threat Detection: Machine learning helps identify potential cybersecurity threats based on unusual activities in network traffic or user behavior.
Automated Incident Response: When security threats are detected, machine learning systems can automatically initiate countermeasures, reducing response times.
Machine learning enhances the ability of organizations to safeguard sensitive data and prevent financial fraud efficiently. By using dynamic, adaptive models that continuously learn from new data, ML systems are better equipped to counter emerging threats compared to static rule-based security systems. This proactive approach significantly reduces the likelihood of successful attacks and helps protect consumer trust in digital financial systems.
6. Advanced Robotics and Automation
Machine learning is driving advanced robotics to perform increasingly complex tasks in industries ranging from manufacturing to healthcare. Robots equipped with ML capabilities are becoming more autonomous, learning from their environments to complete tasks without direct human intervention.
Applications:
Manufacturing Robots: Robots that can adapt to new tasks or optimize processes based on real-time data are improving productivity in factories.
Surgical Robots: ML-powered robots assist in surgeries, offering precision that reduces the risk of human error.
Drones: Machine learning allows drones to navigate independently for delivery, environmental monitoring, and industrial inspections.
Robotics combined with machine learning is reshaping industries by improving efficiency and safety. By enabling robots to adapt to variable conditions and learn from previous experiences, machine learning ensures that these machines can perform tasks in unpredictable environments, paving the way for greater operational flexibility. This evolution in robotics is particularly impactful in hazardous environments, where autonomous systems reduce human risk while increasing efficiency.
7. Predictive Analytics for Business Decision-Making
Businesses rely heavily on predictive analytics to make smarter decisions. ML models analyze past data to forecast trends, identify opportunities, and mitigate risks. This data-driven approach helps companies optimize their operations and stay competitive.
Applications:
Sales Forecasting: Predictive models help businesses forecast demand, enabling better inventory management and sales strategies.
Customer Insights: ML analyzes customer behavior to predict trends and preferences, enabling personalized marketing and product recommendations.
Risk Management: Financial institutions use predictive models to assess potential risks in loans, investments, and market trends.
Predictive analytics gives businesses an edge by enabling proactive decision-making. For example, retail companies use ML to predict when demand for certain products will rise, allowing them to adjust their inventory accordingly. The ability to foresee market changes, customer preferences, or operational inefficiencies means businesses can take action before issues arise, minimizing risks and maximizing profits.
8. Recommendation Engines for E-Commerce and Streaming Services
Machine learning recommendation engines have become incredibly effective in personalizing user experiences. Whether you're shopping online or watching movies, ML analyzes your preferences to suggest products, shows, or content that you're most likely to enjoy.
Applications:
Personalized Shopping: E-commerce platforms use ML to recommend products based on past purchases, browsing behavior, and user preferences.
Streaming Services: Platforms like Netflix and Spotify use recommendation engines to suggest movies, shows, and music that align with your tastes.
Social Media Feeds: Algorithms curate content and advertisements based on user behavior, keeping users engaged longer.
These personalized experiences enhance user satisfaction and increase sales or engagement for businesses. By fine-tuning these recommendation systems, companies can not only enhance user engagement but also drive higher conversion rates, increase customer loyalty, and provide a more enjoyable experience overall. This personalized approach to content and product offerings is becoming a key differentiator in highly competitive markets.
9. Climate Science and Environmental Monitoring
Machine learning is playing a crucial role in addressing climate change and improving environmental monitoring. By analyzing vast amounts of environmental data, ML helps scientists predict climate trends and develop sustainable solutions.
Applications:
Weather Prediction: ML models predict long-term climate patterns and extreme weather events with greater accuracy.
Environmental Monitoring: Real-time analysis of air and water quality helps governments and organizations make informed decisions about environmental protection.
Renewable Energy: Machine learning optimizes the output of renewable energy sources, such as wind and solar power, by predicting energy needs and weather conditions.
Machine learning empowers researchers and policymakers to take more effective actions to protect the planet. By providing actionable insights based on large datasets, ML models are instrumental in devising strategies to mitigate the effects of climate change. This has led to improved decision-making around energy consumption, pollution control, and resource management, contributing to more sustainable practices on a global scale.
10. AI-Powered Education and Learning Platforms
The education sector is being transformed by AI-powered learning platforms that offer personalized learning experiences for students. Machine learning algorithms analyze student progress and adapt educational content to suit individual learning styles, making education more accessible and effective.
Applications:
Adaptive Learning Platforms: AI platforms provide customized learning paths, adjusting difficulty levels and resources based on student performance.
Virtual Tutors: AI-driven tutoring systems offer real-time feedback and support, helping students understand concepts better.
Gamified Learning: Machine learning is used to develop interactive, game-like learning experiences that engage students more effectively.
AI is revolutionizing education by making learning more personalized and interactive, leading to improved educational outcomes. By addressing the unique learning pace and style of each student, AI ensures that no one falls behind, thereby reducing gaps in education quality. The use of virtual tutors allows for a more accessible form of individualized support, which can be particularly beneficial in remote or underserved areas. Gamified learning keeps students engaged, transforming educational environments into dynamic spaces where learning is an exciting and rewarding experience.
Conclusion
Machine learning's transformative potential spans multiple sectors, from healthcare and education to autonomous vehicles and environmental protection. By leveraging advanced algorithms, industries are enhancing efficiency, providing personalized experiences, and addressing complex challenges with innovative solutions. As machine learning technology continues to evolve, its applications are expected to expand even further, shaping the future of how we live and work.
Embracing these innovations allows businesses, governments, and individuals to adapt to new challenges and make the most out of technology’s ever-growing capabilities. As we move forward, machine learning will continue to provide groundbreaking solutions that push the boundaries of what is possible, offering unprecedented opportunities to make our lives more efficient, intelligent, and sustainable.